Conversion Rate Optimization (CRO) is the art and science of strategically improving your website to entice more visitors to take that desired action. It’s about understanding your customers’ behavior, identifying pain points, and implementing changes that guide them toward conversion.
Website Design & Performance = User Experience & Conversions
A slow-loading, clunky website can send potential customers fleeing, while a well-designed, intuitive site can keep them engaged and guide them toward making a purchase.
CRO is key to unlocking your e-commerce success. With the right tools and strategies, you can transform your website into a conversion powerhouse.
Understanding Your E-commerce Conversion Funnel
If you want to optimize your conversions, you need to understand the journey your customers take before they make a purchase. This journey is often visualized as a funnel, where a large number of potential customers enter at the top, and a smaller percentage make it all the way through to the bottom, where they complete a purchase.
The Five Stages of the E-commerce Conversion Funnel
- Awareness: This is the top of the funnel, where potential customers first become aware of your brand and products. They might discover you through search engines, social media, or advertising.
- Interest: Once customers are aware of you, they start to develop an interest in your offerings. They might browse your website, read product descriptions, or watch videos.
- Consideration: At this stage, customers are actively considering whether or not to make a purchase. They might compare your products with competitors, read reviews, or check out your prices.
- Conversion: This is the moment of truth when a customer decides to make a purchase. They add items to their cart and complete the checkout process.
- Retention: Even after a customer makes a purchase, the journey isn’t over. The retention stage is about keeping customers engaged and encouraging them to make repeat purchases.
Analyzing Your Conversion Funnel to Identify Weak Points
Understanding each stage of your funnel is crucial, but it’s equally important to identify where customers are dropping off. This is where funnel analysis comes in. By tracking customer behavior at each stage, you can pinpoint where you’re losing potential sales.
For example, if your homepage has a high bounce rate, your website isn’t immediately grabbing visitors’ attention. If you have a lot of abandoned carts, it could indicate a problem with your checkout process.
Using Data to Drive Your CRO Efforts
Data is your best friend when it comes to CRO. By tracking key metrics like website traffic, bounce rate, cart abandonment rate, and conversion rate, you can gain valuable insights into what’s working and what’s not.
Tools like Google Analytics and Hotjar can help you collect this data and visualize it in an easy-to-understand way. With this information, you can make data-driven decisions about optimizing your website for better conversions.
Heatmaps and User Behavior Analysis: Seeing Through Your Customers’ Eyes
Heatmaps are visual representations of how users interact with your website. They can show you where users are clicking, how far they’re scrolling, and where they’re spending the most time. This information can be invaluable for identifying potential problems and areas for improvement.
For example, if you see that users are clicking on a button that doesn’t work, you can quickly fix it. Or, if you notice that users are abandoning their carts at a certain point, you can investigate why and make changes to streamline the checkout process.
Elementor: Your All-in-One CRO Toolkit
Elementor isn’t just a website builder; it’s also a powerful CRO tool. With its built-in analytics and heatmap integrations, you can easily track user behavior and identify areas for improvement. Plus, Elementor’s intuitive drag-and-drop interface makes it easy to experiment with different layouts and designs to see what works best for your audience.
Grow Your Sales
- Incredibly Fast Store
- Sales Optimization
- Enterprise-Grade Security
- 24/7 Expert Service
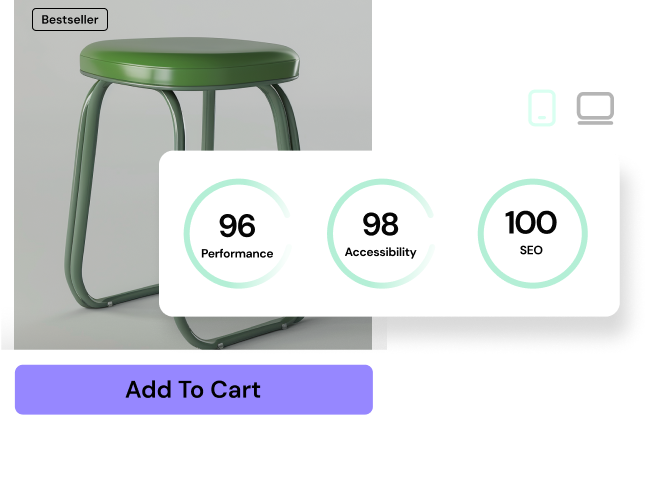
- Incredibly Fast Store
- Sales Optimization
- Enterprise-Grade Security
- 24/7 Expert Service
- Prompt your Code & Add Custom Code, HTML, or CSS with ease
- Generate or edit with AI for Tailored Images
- Use Copilot for predictive stylized container layouts
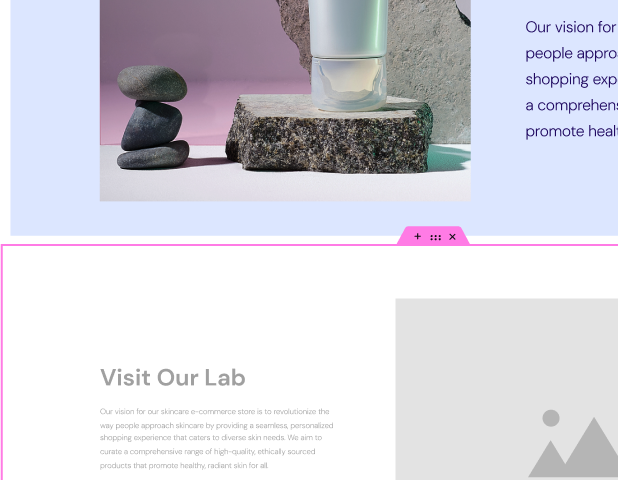
- Prompt your Code & Add Custom Code, HTML, or CSS with ease
- Generate or edit with AI for Tailored Images
- Use Copilot for predictive stylized container layouts
- Craft or Translate Content at Lightning Speed
Top-Performing Website
- Super-Fast Websites
- Enterprise-Grade Security
- Any Site, Every Business
- 24/7 Expert Service
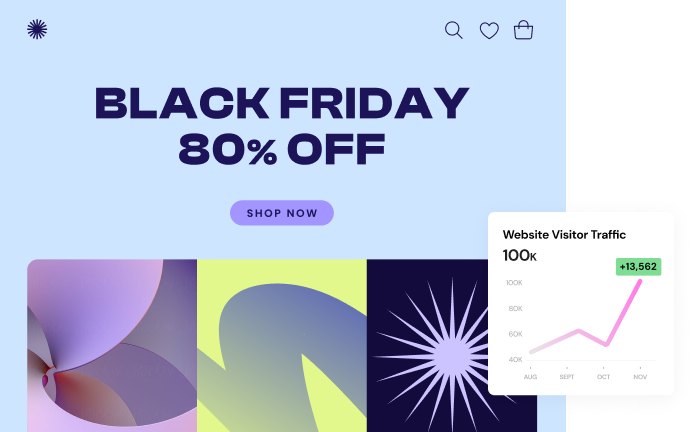
Top-Performing Website
- Super-Fast Websites
- Enterprise-Grade Security
- Any Site, Every Business
- 24/7 Expert Service
- Drag & Drop Website Builder, No Code Required
- Over 100 Widgets, for Every Purpose
- Professional Design Features for Pixel Perfect Design
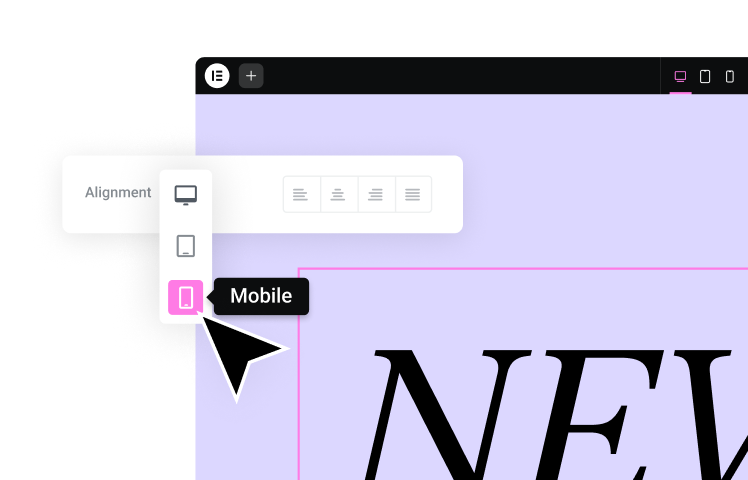
- Drag & Drop Website Builder, No Code Required
- Over 100 Widgets, for Every Purpose
- Professional Design Features for Pixel Perfect Design
- Marketing & eCommerce Features to Increase Conversion
- Ensure Reliable Email Delivery for Your Website
- Simple Setup, No SMTP Configuration Needed
- Centralized Email Insights for Better Tracking
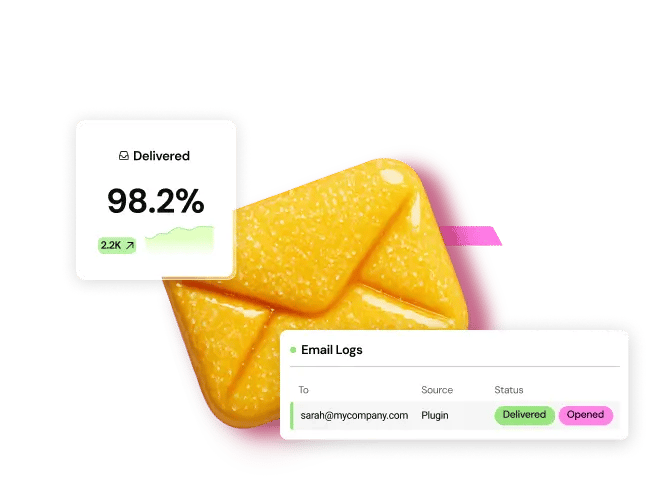
- Ensure Reliable Email Delivery for Your Website
- Simple Setup, No SMTP Configuration Needed
- Centralized Email Insights for Better Tracking
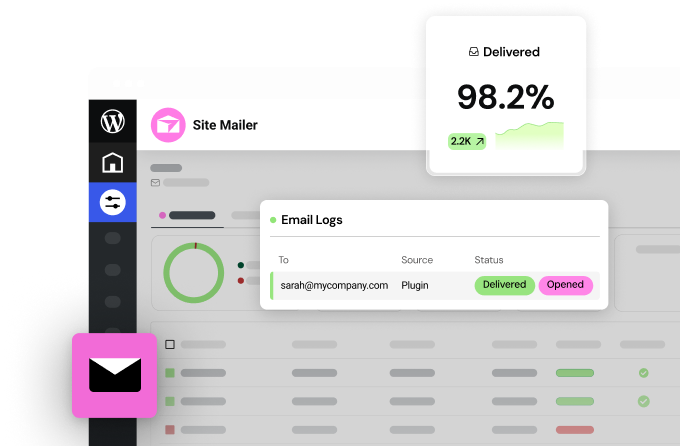
- Ensure Reliable Email Delivery for Your Website
- Simple Setup, No SMTP Configuration Needed
- Centralized Email Insights for Better Tracking
Crafting High-Converting Landing Pages
Your landing pages are often the first impression customers have of your brand. Whether they arrive from a search engine result, a social media ad, or an email campaign, these pages need to be optimized to capture attention, communicate value, and drive conversions.
The Essential Elements of a High-Converting Landing Page
- A Crystal-Clear Value Proposition: Visitors should understand what you’re offering and why it’s valuable to them within seconds of landing on your page. Your value proposition should be concise, compelling, and prominently displayed.
- A Compelling Headline: Your headline is the first thing visitors will read. It should be attention-grabbing, relevant to the traffic source, and clearly communicate the benefit of your product or service.
- A Strong Call to Action (CTA): Your CTA tells visitors what you want them to do—buy now, sign up, download, etc. Make your CTA stand out with contrasting colors, persuasive language, and prominent placement on the page.
- Persuasive Copywriting: The copy on your landing page should be clear, concise, and benefit-oriented. Focus on how your product or service solves your customers’ problems and makes their lives better.
- Social Proof: Testimonials, reviews, case studies, and trust badges can all help build credibility and encourage visitors to convert.
- A Sense of Urgency: Limited-time offers, countdown timers, and low-stock notifications can create a sense of urgency and encourage visitors to take action before it’s too late.
Visual Appeal: More Than Just Pretty Pictures
Your landing page’s visual design plays a crucial role in conversion. High-quality images, videos, and an overall aesthetic that aligns with your brand can significantly impact how visitors perceive your business.
Images: Use professional, high-resolution photos that showcase your products in the best possible light. Consider using lifestyle images that show your products to help customers visualize how they can benefit from them.
Videos: Product demos, explainer videos, and customer testimonials can add a dynamic element to your landing pages and help you connect with your audience on a deeper level.
Design: Your landing page design should be clean, uncluttered, and easy to navigate. Use a consistent color scheme, typography, and visual hierarchy to guide visitors’ attention to the most important elements.
Mobile Optimization: Catering to the On-the-Go Shopper
Your landing pages must be optimized for mobile devices. A responsive design that adapts to different screen sizes is a must. Make sure your text is readable, your buttons are easy to tap, and your overall design is user-friendly on smaller screens.
Consider using mobile-specific features like click-to-call buttons and location-based services to make it even easier for mobile users to engage with your brand.
A/B Testing: The Scientific Approach to CRO
A/B testing (or split testing) involves creating two or more versions of a landing page and comparing their performance. By testing different headlines, CTAs, images, layouts, and other elements, you can identify which version performs best and make data-driven decisions to improve your conversion rate.
With Elementor, you can easily create multiple variations of your landing pages and run A/B tests to see what resonates best with your audience. Its intuitive interface and built-in testing capabilities make it simple to experiment and optimize.
Image Recognition and Computer Vision
Machine learning has revolutionized image recognition and computer vision, enabling machines to interpret and understand visual information from the world around us. This field encompasses a wide range of applications, from simple object detection and classification to complex scene understanding and facial recognition.
Tasks in Image Recognition and Computer Vision
Image recognition and computer vision involve various tasks, each addressing different aspects of visual information processing:
- Image Classification: This fundamental task involves assigning a label or category to an entire image, such as determining whether it contains a cat, dog, or car.
- Object Detection: A step further than classification, object detection not only identifies objects within an image but also locates them by drawing bounding boxes around them. This is crucial for applications like autonomous driving, where precise object localization is essential.
- Image Segmentation: This task involves dividing an image into meaningful regions or segments, each corresponding to a different object or part of an object. It’s like digitally coloring within the lines to delineate objects from the background and each other. Image segmentation is crucial for applications like medical imaging, where it can be used to identify tumors or organs.
- Object Tracking: Beyond mere detection, object tracking involves following the movement of objects across consecutive video frames. This is vital for video analysis, surveillance, and autonomous systems that need to understand how objects change position over time.
- Pose Estimation involves identifying the position and orientation of specific body parts or objects within an image or video. It has applications in fields like augmented reality (AR), where it can overlay digital information onto the real world, and in healthcare, where it can analyze human movement and posture for rehabilitation or diagnosis.
- Image Generation: While most tasks focus on understanding existing images, image generation involves creating new images from scratch. This can be done using techniques like Generative Adversarial Networks (GANs) or Variational Autoencoders (VAEs). Image generation has applications in art, design, and even data augmentation for training other machine learning models.
Techniques in Image Recognition
A variety of machine learning techniques power image recognition and computer vision tasks:
- Convolutional Neural Networks (CNNs) are the workhorses of image recognition. They are designed to learn hierarchical features from raw image data automatically. Due to their ability to capture spatial relationships within images, CNNs excel at tasks like image classification, object detection, and segmentation.
- Recurrent Neural Networks (RNNs): While CNNs focus on spatial information, RNNs specialize in temporal information. They are often used in tasks like image captioning, where the goal is to generate a textual description of an image, as they can process sequences of data (like words in a caption) and maintain the memory of previous information.
- Transformers: Originally developed for natural language processing, transformers have recently shown promising results in image recognition. Vision Transformers (ViTs) treat images as sequences of patches and use self-attention mechanisms to capture global relationships between different image regions. This enables them to learn complex representations and achieve state-of-the-art performance on various tasks.
- Transfer Learning: Training a deep neural network from scratch can be computationally expensive and require large amounts of labeled data. Transfer learning addresses this by leveraging pre-trained models on massive datasets (like ImageNet) and fine-tuning them on smaller, task-specific datasets. This significantly speeds up training and improves performance, especially when limited data is available.
Applications of Image Recognition
Image recognition has found applications in numerous fields, transforming how we interact with technology and the world:
- Healthcare: In medical imaging, machine learning algorithms can analyze X-rays, CT scans, and MRIs to assist in the detection and diagnosis of diseases like cancer, Alzheimer’s, and diabetic retinopathy. It also enables more efficient and accurate pathology analysis and personalized treatment plans.
- Autonomous Vehicles: Self-driving cars rely heavily on image recognition to identify road signs, pedestrians, other vehicles, and obstacles. These systems interpret real-time camera and sensor data to make critical driving decisions, ultimately aiming to improve road safety and efficiency.
- Security and Surveillance: Facial recognition technology is used in surveillance systems for security purposes, such as identifying individuals in crowds or verifying identities at airports. Image recognition can also be used for object detection in security footage to alert authorities of suspicious activity.
- Retail and E-commerce: Image recognition is transforming the shopping experience. Visual search allows customers to find products by simply taking a picture or uploading an image. Virtual fitting rooms enable customers to try on clothes virtually, while inventory management systems use image recognition to track stock levels and automate product tagging.
- Agriculture: In precision agriculture, drones equipped with cameras and machine learning algorithms can monitor crop health, detect pests and diseases, and optimize irrigation and fertilization. This helps farmers make data-driven decisions to improve yields and reduce costs.
Manufacturing and Robotics: In industrial settings, image recognition is used for quality control, identifying defects and anomalies in products on assembly lines. Robots equipped with computer vision can perform tasks like picking and sorting objects, welding, and even complex assembly operations. - Augmented Reality (AR): Image recognition enables AR applications to seamlessly overlay digital information into the real world. This has applications in gaming (e.g., Pokémon Go), education (interactive textbooks), and even interior design (visualizing furniture in a room).
Social Media and Content Moderation: Image recognition automatically flags and filters inappropriate or harmful content on social media platforms. This is to maintain a safer online environment and protect users from exposure to violence, hate speech, or explicit material. - Accessibility: Image recognition technology can be used to assist visually impaired individuals by generating audio descriptions of images and scenes. This can help them navigate the physical world and access information online more independently.
Ethical Considerations and Challenges in Image Recognition
While image recognition offers tremendous potential, it’s not without ethical considerations and challenges:
- Bias and Fairness: Machine learning models can inherit biases present in the domain on which they are based. This can lead to discriminatory outcomes, such as facial recognition systems performing poorly on individuals with darker skin tones or misidentifying individuals from certain ethnic groups. Addressing bias requires careful data collection, model training, and ongoing evaluation.
- Privacy Concerns: The widespread use of image recognition technology raises privacy concerns. Facial recognition, in particular, can be used for surveillance and tracking without individuals’ knowledge or consent. Striking a balance between security and privacy is crucial to ensure responsible use of this technology.
Misuse and Manipulation: Image recognition technology can be misused to create deepfakes—highly realistic manipulated videos or images that can be used to spread misinformation or defame individuals. Deepfakes pose a significant threat to trust and authenticity in the digital age. - Accountability and Transparency: As image recognition systems become more sophisticated and integrated into critical decision-making processes, the need for transparency and accountability grows. Understanding how these systems make decisions and ensuring they are not used to discriminate or harm individuals is crucial.
- Security Risks: Adversarial attacks, where malicious actors intentionally manipulate images to fool image recognition systems, pose a security risk. These attacks can have serious consequences in applications like autonomous vehicles, where misclassification of objects can lead to accidents.
The Future of Image Recognition
The future of image recognition is bright, with ongoing research and development pushing the boundaries of what’s possible:
- Explainable AI (XAI): As image recognition systems become more complex, understanding their decision-making processes becomes crucial. XAI aims to make these systems more transparent and interpretable, providing insights into why a model makes a particular prediction. This is essential for building trust and ensuring ethical use.
- Real-time Image Recognition: Advances in hardware and software are enabling faster and more efficient image recognition in real time. This opens up possibilities for applications like instant language translation from images, live augmented reality experiences, and more responsive autonomous systems.
- Zero-Shot and Few-Shot Learning: One of the most exciting frontiers in image recognition is the development of models that can recognize objects or perform tasks with minimal or no training examples. Zero-shot learning aims to generalize knowledge from seen categories to unseen ones, while few-shot learning focuses on learning from just a handful of examples. These approaches have the potential to revolutionize how we adapt image recognition systems to new domains and tasks quickly.
- 3D Image Recognition: While most image recognition focuses on 2D images, there’s growing interest in developing models that can understand and interpret 3D data from sources like LiDAR and depth cameras. This has applications in autonomous navigation, robotics, and augmented reality, where understanding the spatial relationships between objects in the real world is crucial.
Multimodal Learning: The integration of image recognition with other modalities like natural language processing and audio analysis opens up a new realm of possibilities. For example, models that can understand both images and text can be used for tasks like visual question answering, where the goal is to answer questions about an image based on its visual content and textual context. - Edge Computing: Processing image data on edge devices like smartphones and IoT sensors can reduce latency, improve privacy, and conserve bandwidth. This is driving the development of specialized hardware and algorithms optimized for image recognition on resource-constrained devices.
Conclusion
Image recognition, powered by machine learning, has emerged as a transformative technology with far-reaching applications across industries. From healthcare and autonomous vehicles to retail, agriculture, and beyond, image recognition is reshaping how we interact with the world and enabling new possibilities. As research continues and the technology matures, we can expect even more innovative and impactful applications in the years to come.
However, it’s essential to navigate the ethical challenges and ensure the responsible development and deployment of image recognition systems. Addressing issues like bias, privacy, and misuse will be critical to harnessing the full potential of this technology for the benefit of society.
Looking for fresh content?
By entering your email, you agree to receive Elementor emails, including marketing emails,
and agree to our Terms & Conditions and Privacy Policy.